Generative AI (GenAI) models like GPT and Google’s BERT are trained on vast datasets that enable advanced language comprehension, contextual understanding, and sensitivity to nuanced details. These models have been developed using extensive resources collected from a diverse array of publicly available sources, including websites, forums, books, and other digital repositories. As technology progresses, developers face an increasing challenge in sourcing substantial quantities of fresh data to match the sophistication of the advanced models they create.
Diminishing Data Returns
Source: Exploding Topics
When data is repeated or similar sources are reused, overfitting can occur, causing the AI to become overly specialized to its training set, which reduces its effectiveness in novel or unpredictable situations. However, as we approach the limits of data expansion, it becomes evident that these models risk becoming static or losing sophistication.
What AI Firms Are Saying: Voices from OpenAI, Google, and Beyond
As concerns about data scarcity grow, leaders in AI have begun sharing their perspectives:
- OpenAI: OpenAI researchers are tackling the issue of data quality deterioration and are advocating for a shift towards smaller, more focused datasets. They believe that training general-purpose models on vast, unrestricted datasets may become impractical. Instead, they suggest that smaller datasets tailored to specific industry objectives may prove more effective.
- Google DeepMind: Google has emphasized creating models designed for specialized applications. For example, its Gemini project explores integrating diverse data types—such as text, images, and video—into a unified model to maximize the utility of existing data resources.
- Meta: Mark Zuckerberg’s Meta is pursuing a different approach, focusing on models like Llama, which excel in specific tasks using existing data. Meta’s current models prioritize performance and efficiency, relying on smaller, targeted datasets during training.
Source: Meta
These perspectives collectively suggest a potential shift from large, general-purpose language models toward more specialized AI systems designed for targeted audiences or specific applications. This approach enables data to be utilized more intelligently and efficiently, maximizing relevance and effectiveness.
Consequences of Data Scarcity for AI Quality
With restricted access to novel data, GenAI’s progress may experience several potential effects:
- Decreased Accuracy and Relevance: Models without updated information are exposed to filling gaps in knowledge which they will be unable to provide accurate intelligence. The world has a degree of dynamism and so do its languages, trends, and knowledge bases. A language model primarily trained with 2020 data may lack comprehension of contemporary occurrences thereby resulting in irrelevant or erroneous feedback.
- Increased Homogeneity in Responses: A continuous application of only the same datasets can lead to a situation where the outputs of the AI are overly predictable. There’s a risk of repetitiveness in response to issues, which negates the novelty and variety in the deployment of generative materials, for instance, when the model is used in creative writing, customer care, or during content creation.
- Shift Toward Narrow AI Models: Since data is becoming limited, companies may turn to developing narrow AI models focused on specific products rather than developing general-purpose models that are quite versatile. Though narrow AI models are very powerful within their context, the possibility of this paradigm shift might limit the reach that AI was believed to assist.
The Next Frontier: Alternatives to Traditional Data Sources
To combat the scarcity issue, AI firms and researchers are exploring several approaches:
Synthetic Data Generation
Synthetic data, or data generated by AI, offers a promising solution to this challenge. These datasets, created through simulations or augmentations of real data, are particularly valuable in fields requiring privacy-protected or domain-specific data.
However, synthetic data comes with its own limitations. Models may perpetuate the biases present in the original data, potentially leading to inaccuracies and reinforcing biased perspectives.
Human-in-the-Loop (HITL) Training
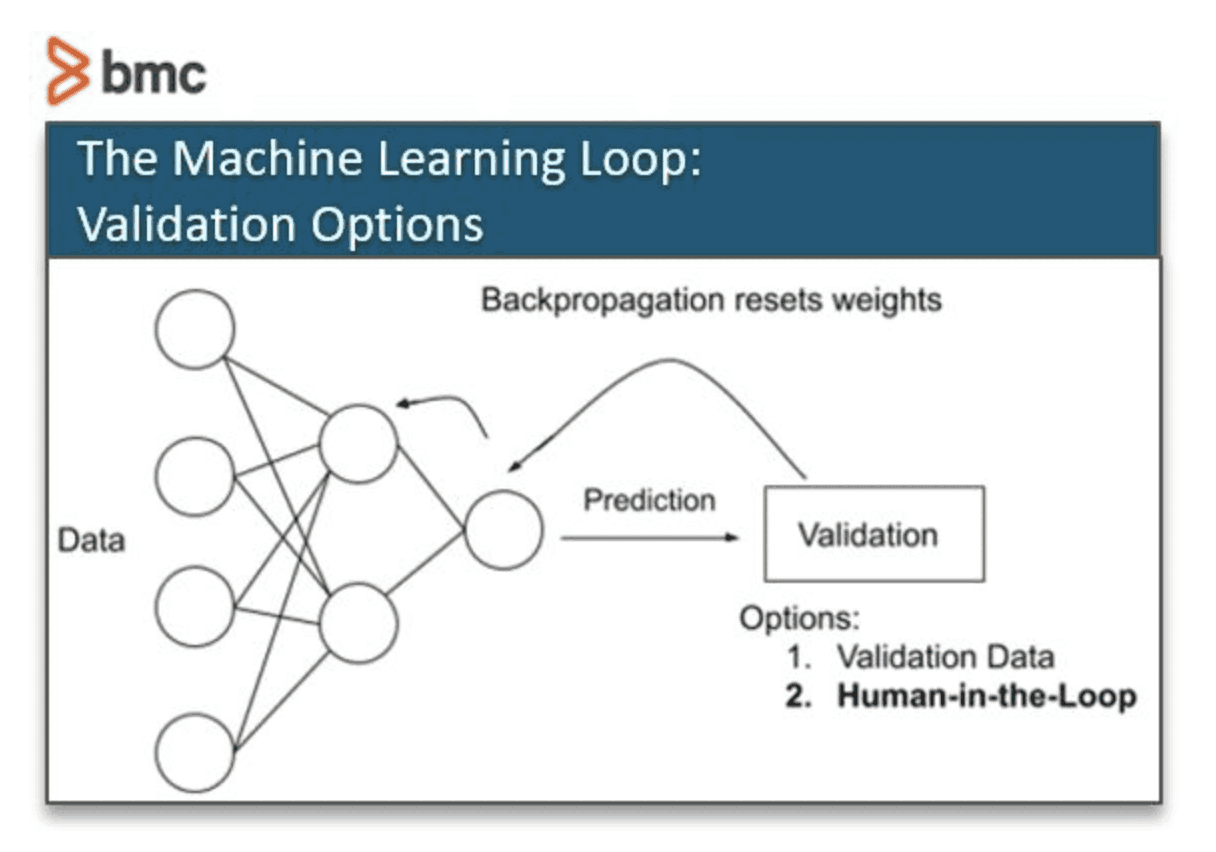
Federated learning decentralizes data by training AI models across multiple endpoints, such as phones or computers, without consolidating vast datasets in a single location. This technique, increasingly popular in personal device AI, offers the potential to generate large-scale insights without relying on traditional data acquisition methods.
Data Partnerships and Licenses
Data licensed from organizations, universities, and private companies could eventually support AI companies in acquiring very specific and up-to-date datasets. However, such agreements also prompt cost, accessibility, and ethical concerns in cases of private or sensitive data.
The AI Data Hunger Games: Ethics on the Chopping Block?
AI, much like Katniss in The Hunger Games, raises a crucial question: where will the ethical boundaries be drawn?
Privacy Compromised: Consent—An Afterthought?
In the relentless pursuit of data, companies may transform into digital scavengers, often disregarding fair use and scraping virtually any available content. Imagine an AI company as a “copyright ninja,” skirting designers’ rights and ethical regulations with ease. This unchecked data acquisition leaves individuals and businesses exposed to privacy violations and a potential minefield of legal entanglements.
Copyright Conundrum: Innovation or Infringement?
AI models are trained on massive data troves—books, media, websites, and more. But where does borrowing end and overreach begin? Recent lawsuits against OpenAI and Google for allegedly using people’s images to ‘identify’ them underscore rising concerns. Given current laws and market practices, the AI industry is likely heading into a period of intense legal scrutiny, potentially relying on prolonged litigation to defend its practices. The outcome may well leave a lasting impact on reputations and industry ethics.
Source: James Grimmelmann
Synthetic Data: A Double-Edged Sword
While synthetic data offers a glimmer of promise, it brings forth a pressing question: if the data is generated, how can we ensure it remains untarnished by bias? This creates a complex cycle: an AI model trained on biased data can inadvertently embed those same biases in the synthetic data it produces. This raises concerns about sectors like recruitment, law enforcement, and healthcare potentially becoming arenas of discrimination—mediated by biased algorithms. To avoid such a dystopian future, we must establish robust ethical guidelines for synthetic data generation, ensuring fairness and neutrality.
The potential of AI is immense, but it comes with significant ethical costs. A comprehensive regulatory framework is essential to prevent AI from evolving into a ravenous, unchecked entity that disregards privacy, copyright, and fairness.
The issue goes beyond mere legal compliance—it is about fostering trust and ensuring that AI genuinely benefits humanity.
How Regulators Are Responding to the Data Shortage Challenge
Governments around the world are grappling with the implications of AI’s insatiable demand for data:
- European Union’s AI Act: The European Union has taken a leading role in AI regulation through its AI Act, which emphasizes transparency, data protection, and accountability. The EU’s approach to regulating data sourcing and AI practices could set a precedent for other countries as they develop their own frameworks.
- U.S. Federal Trade Commission (FTC): In the U.S., the FTC is closely monitoring AI practices to curb deceptive or unfair activities. The commission is scrutinizing AI companies’ data collection, storage, and usage practices, particularly when sensitive data is involved.
- UNESCO’s AI Ethics Recommendation: UNESCO’s guidelines promote ethical AI practices by advocating for transparency, accountability, and non-discrimination. While these guidelines are not legally binding, they reinforce the global push for ethical and inclusive AI development.
What’s Next? Adapting AI Development in a World with Limited Data
Source: Viso
- Emphasis on Interdisciplinary Collaboration: AI companies may increasingly collaborate with experts in fields requiring nuanced understanding, enhancing both the depth and scope of AI outputs without excessive reliance on new data. Such partnerships could help refine models with insights from specialized domains, boosting AI’s relevance and accuracy.
- Strengthened AI Regulations and Compliance Practices: With data scarcity intensifying, AI companies may ramp up efforts to source data more aggressively. However, this pressure could also prompt governments to reinforce regulations around data sourcing, usage, privacy, and intellectual property, ensuring responsible AI development.
- Public-Private Data Initiatives: As countries expand their AI ambitions, they may encourage public-private partnerships in sectors like healthcare, education, and environmental science. These collaborations could facilitate data sharing between public institutions and AI companies, creating a mutually beneficial ecosystem that accelerates innovation in critical areas.